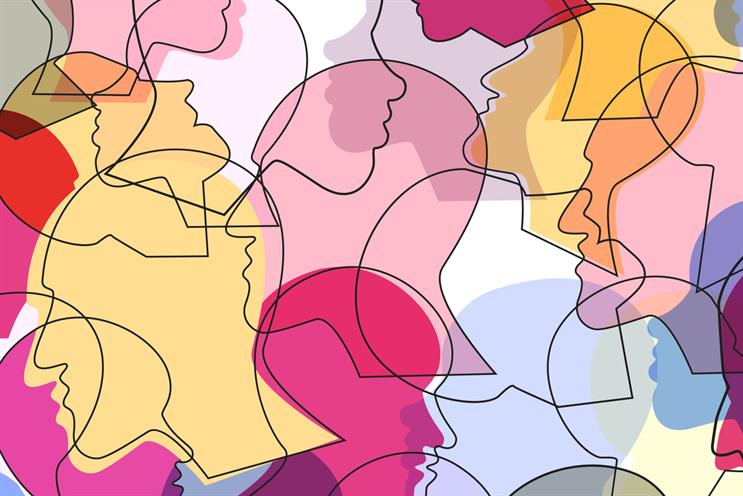
How do you go about exploring Twitter in the UK? How could you uncover the communities that live and thrive on it? It is a daunting yet hugely exciting task.
And that’s exactly what we set out to do earlier this year. We wanted to find out about the individuals behind the Tweets – uncover motivations, likes, dislikes and discover exactly who makes up our different communities.
Our journey to dig deeper
We needed an approach that would enable us to go broad and deep to capture Twitter’s unique nature. This meant we had to push the boundaries of standard data science. We partnered with Jaywing Intelligence to design an approach; it started with our data to uncover stable communities on Twitter that we could dig into further.
Think of Twitter as an unimaginably vast room, a room that’s so big it can fit everyone in the world in – and more.
In this infinite room, people talk to each other and start to "self-organise" based on shared interests. This is similar to when you go to a party you end up gravitating towards people who are talking about stuff you’re into.
Now imagine you’re entering the room and don’t know anyone. Where do you go and who do you speak to? It’s hard to know.
Fortunately, this room has many screens on the wall showing content that might interest you. You head to the screen showing the content you’re interested in and you get chatting to other people who like that content.
You start to build connections with those around you. Slowly the content becomes secondary to the conversations you’re having with the people. When the content stops the conversation and the connection continues. Over time, groups grow existing independently of content.
It’s these persistent communities we wanted to find, the ones that exist above and beyond a particular trend or moment.
Kings of infinite space
So if you think of the infinite room as Twitter, how do we go about finding communities? In this case, we have to look beyond Tweets alone into follower graphs and bios of over four million UK accounts to find these communities with any confidence.
Once we had established a method to uncover persistent communities, there were two considerations: how do we make sure these communities aren’t random; how do we know that they matter enough for us to care?
Using mathematics and data from the Twitter eco-system, we could identify the persistent communities and ignore the random. This is based on p-values. Once we’d found the persistent communities, the ones that don’t just form randomly, our task was to understand... do they matter? To address this, we looked at two dimensions for every community: size and density.
Size is pretty straight forward: how large the communities uncovered were, how many accounts make them up. Density is the second dimension – how interconnected is the community?
And we found some interesting stuff – who knew there was a Cheese Twitter? Or that police and nurses use Twitter in a similar way, where teachers use it to share best practice?
Starting with Twitter data allowed us to find things we didn’t know were there.
But we also honed in on four communities that appeared to have the biggest presence on Twitter – football, gaming, music and health.